Analysis of facial expressions of emotions in children
The study described in this guest blog post focuses on the facial expressions of emotions induced by affective stimuli in children aged between 7 and 14.
Posted by
Published on
Thu 14 Feb. 2019
Topics
| Action Units | Emotion Recognition | Emotions | FaceReader | FACS |
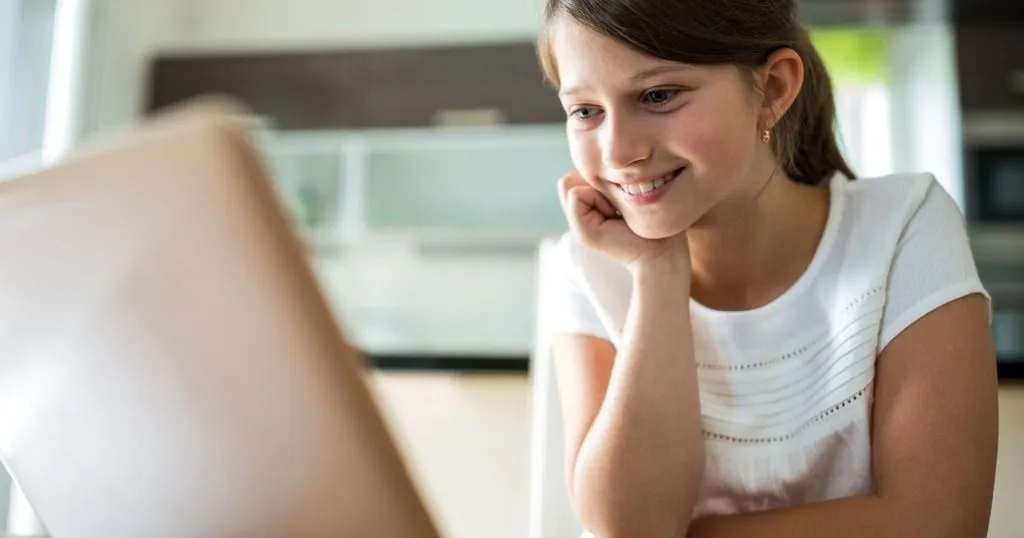
In this guest blog post, researchers involved in the FIRB and NARSAD projects from the Vita-Salute San Raffaele University and the Sigmund Freud University in Milan, Italy, tell us more about their study carried out to characterize a set of emotionally charged stimuli in terms of the induced facial activation. In the project, FaceReader along with a FACS coder were used to investigate the differences between the two.
The team consists of: Dr. Regina Gregori Grgič (Vita-Salute San Raffaele University, Milano); Dr. Simona Scaini (Sigmund Freud University, Milano); Dr. Chiara Ferrero (Vita-Salute San Raffaele University, Milano); Dr. Valentina Latronico (Unit for Cognitive Neuroscience (UCN), Department of Psychology, University of Copenhagen, Denmark); Prof. Anna Ogliari (Vita-Salute San Raffaele University, Milano); Prof. Clelia Di Serio (Vita-Salute San Raffaele University, Milano); Dr. Chiara Brombin (Vita-Salute San Raffaele University, Milano).
Analysis of facial expressions of emotions in children
The present study has been developed within the activities of a larger project1 aimed at measuring emotional response by integrating different sources of information such as facial expressions, physiological measurements, psychometrics, and clinical data. Here, we focus on the facial expressions of emotions induced by affective stimuli in children taken from the general population.
Using Facial Action Coding System (FACS) for emotion recognition
Why facial expressions? And why children? The early identification of deficits in emotion recognition and expression skills may prevent poor social functioning in adulthood. Moreover, facial expressions represent the most effective way to convey information on one’s emotional state to others.
Using the Facial Action Coding System (FACS; Ekman and Friese, 1978), facial configurations may be decoded using specific, quantitative, and sharable rules. Facial expressions are described in terms of single movements of face muscles (Action units, AUs) and their intensity.
FREE WHITE PAPER: Facial Action Coding System
Download here the FREE white paper 'Facial Action Coding System (FACS)' and learn more about:
- Objective results with FACS
- Action Units
- Examples using FACS
Measuring facial expressions of children
To elicit emotions, we used a pre-tested set of 15 images from the Anger and Fear Eliciting Stimuli for Children (AFES-C, Scaini et al., 2017), specifically suited to elicit anger, fear, and neutral emotions. These emotions are actually linked with the development of both internalizing and externalizing problems.
A group of 61 children aged between seven and fourteen took part in the experimental sessions, which were carried out in a laboratory. The children’s facial expressions were recorded during the stimuli administration. No instruction was given to display a specific emotion, so the recording attested to the spontaneous reaction of the children. Lighting conditions were carefully controlled.
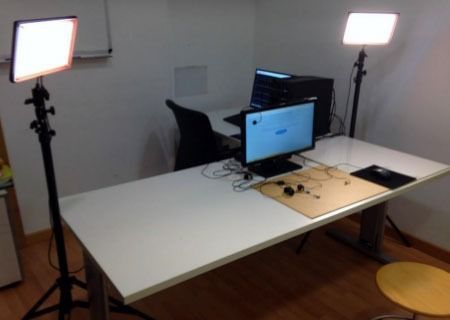
Figure 1: The lab setup.
Emotional responses
After each stimulus presentation, children were asked to describe their emotional experience using both the discrete and dimensional models of emotions. Children were allowed to move and assume natural poses, thus making the analysis of their facial expressions more difficult for the software. No real-time analysis of the videos was performed.
The goal of the analysis was to characterize emotional response induced by the AFES-C stimuli using facial expression data recorded by FaceReader and the certified FACS human coder. The idea was to identify patterns of AUs specific to each target emotion.
Automatic detection of Action Units versus a FACS coder
The most frequently detected AUs by FaceReader were 43 (eyes close), 17 (chin raise) and 25 (mouth open); the least detected were 9 (curl nose), 20 (pull the chin back) and 27 (stretch mouth).
The FACS coder detected some AUs as more frequent, including the AUs 4 (wrinkle eyebrow), 5 (dilate eyes) and 1 (wrinkle front). The AUs that were the least detected were AUs 20, 43 and 27. Results are shown in Figure 2.
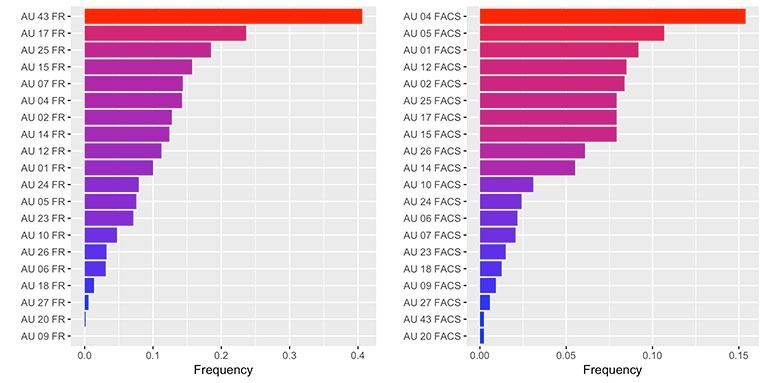
Figure 2: Barplot showing the relative frequency of the occurred Action Units, detected by FacerReader 5 (on the left) and by the FACS coder (on the right), in decreasing order. When considering FaceReader, the most frequently detected AU was AU43, indicating “eyes closed”, while the FACS coder detected AU4 with the highest frequency, indicating “brow lowerer”. The least detected AU with Facereader was AU9 (nose wrinkler), while for the FACS coder it was AU 20 (lip stretcher).
Since the occurrence of AUs for the FACS coder is a binary variable, to compare the two methods, the continuous AU signal provided by FaceReader was dichotomized using an empirical threshold: the AU occurred if the maximum of the signal was larger than 0.2 (in the 0-1 range) at least once throughout the stimulus presentation time.
Clustering techniques were then applied on the variables indicating the occurrence of the AUs detected by both the automatic and the manual method. In particular, we focused on the most emotion-relevant AUs, e.g., on the AUs 1 (raise eyebrows), 4, 5, 7 (tighten the eyes), 10 (pull on cheeks), 12 (smile), 14 (dimples), 15 (corners of the mouth down), 17 (chin upward), 20, and 23 (stiffen lips). Hence, we excluded AUs which were never or always detected by the two methods, e.g., the 27 and the 43.
When considering AUs detected by FaceReader, we identified two clusters of images, including respectively neutral and non-neutral stimuli; when considering AUs detected by the FACS coder, three clusters, almost reflecting the three target emotions, were identified.
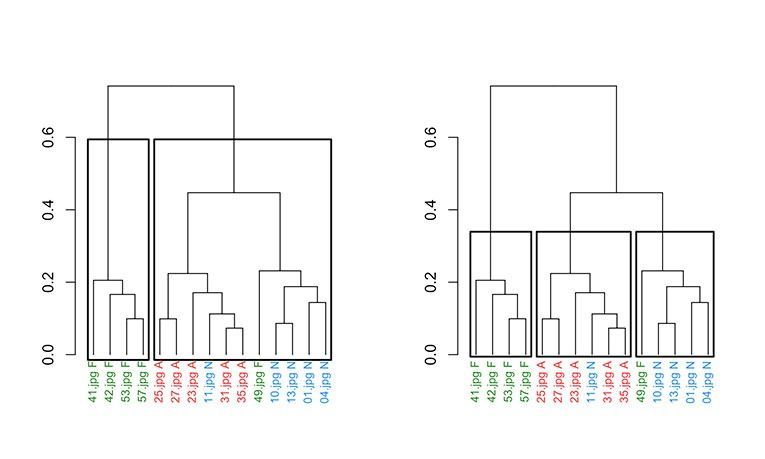
Figure 3: Clustering stimuli based on the Action Units detected by FacerReader 5 (on the left) and by the FACS expert (on the right). Each picture is labelled with its ID. N denotes neutral pictures, anger-eliciting pictures are indicated by the letter A, and finally letter F is associated to fear- inducing pictures.
Experience with FaceReader
Exploring FaceReader 5 capabilities in an experimental setting involving children was very interesting. Also, in our study we were interested in natural, spontaneous facial expressions. In some cases, FaceReader failed to detect a child’s face, due to the size of the face itself, movements of the child, or the presence of glasses/hair causing partial occlusions. We used static stimuli without inducing strong emotional responses, but whenever the child expressed a stronger emotional facial display, the software successfully analyzed the facial expression.
We found that the “General” model of FaceReader performed better than the “Children” model (Loijens and Krips, 2018). We are aware that the definition of AU “occurrence” based on the 0.2 empirical threshold could affect our results, and we are currently refining the definition of this threshold using functional analysis techniques.
The latest version of FaceReader actually uses a new deep learning algorithm, called Deep Face Model, allowing you to analyze facial expressions under challenging conditions. Hence, as matter of future work, we would like to test its performance in the same experimental setting.
FREE WHITE PAPER: FaceReader methodology
Download the free FaceReader methodology note to learn more about facial expression analysis theory.
- How FaceReader works
- More about the calibration
- Insight in quality of analysis & output
References
- Ekman, P., Friesen, W. V. (1978). “Manual for the facial action coding system”. Consulting Psychologists Press.
- Loijens, L., Krips, O. (2018). “FaceReader Methodology Note”. Behavioral research consultants at Noldus Information Technology.
- Scaini, S., Rancoita, P. M., Martoni, R. M., Omero, M., Ogliari, A., & Brombin, C. (2017). Integrating Dimensional and Discrete Theories of Emotions: A New Set of Anger-and Fear-Eliciting Stimuli for Children. The Journal of genetic psychology, 178 (5), 253-261.
1 This research has been funded by the FIRB Project RBFR12VHR7 “Interpreting emotions: a computational tool integrating facial expressions and biosignals based on shape analysis and bayesian networks” (PI, Dr. Brombin) and by a NARSAD Young Investigator Grant from the Brain & Behavior Research Foundation, grant number: 23553, entitled “Integrating multimodal affective information for emotion recognition in children with symptoms of anxiety disorders” (Dr. Scaini recipient).
Related Posts
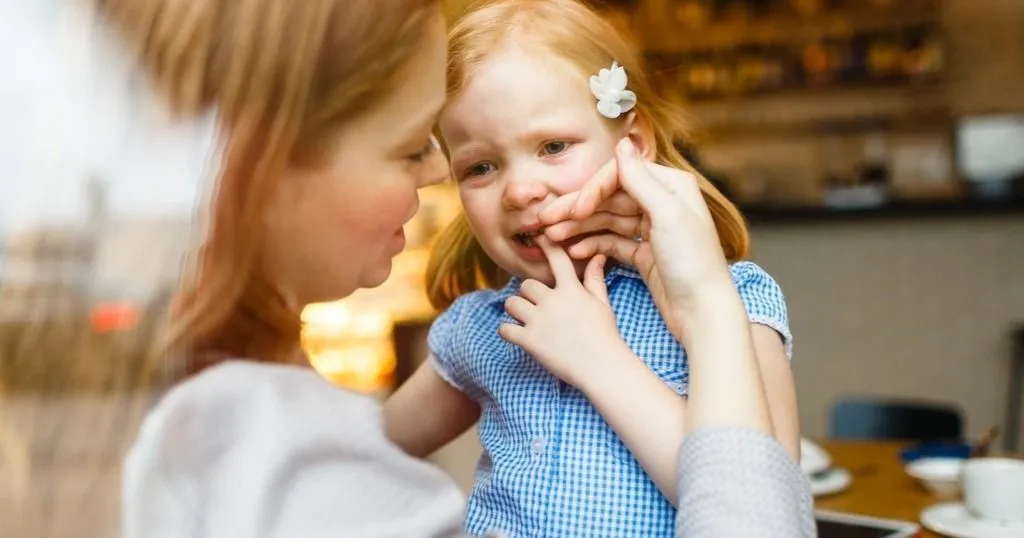
Emotional responses to infant crying
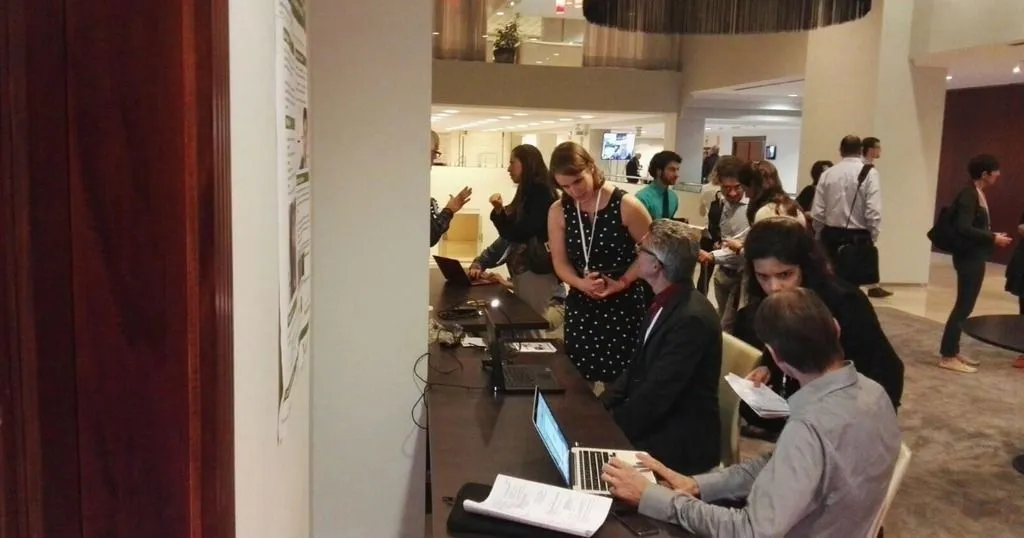
Technical demonstration of FaceReader in Seville, Spain
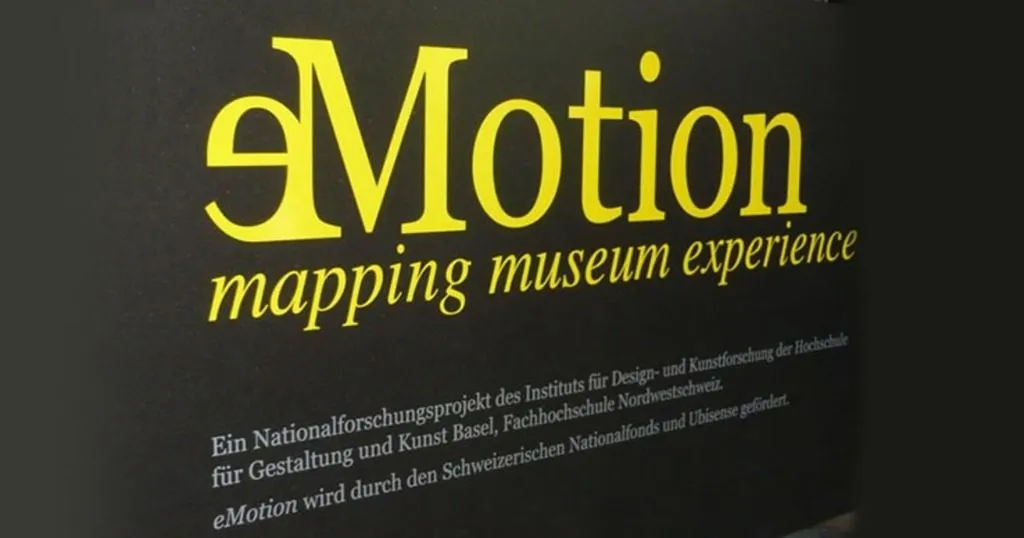