Comparing machine emotion recognition with a human benchmark
Our emotions come across clear in our facial expressions. Due to this, facial expressions can be used in a wide variety of studies.
Posted by
Published on
Fri 06 Sep. 2013
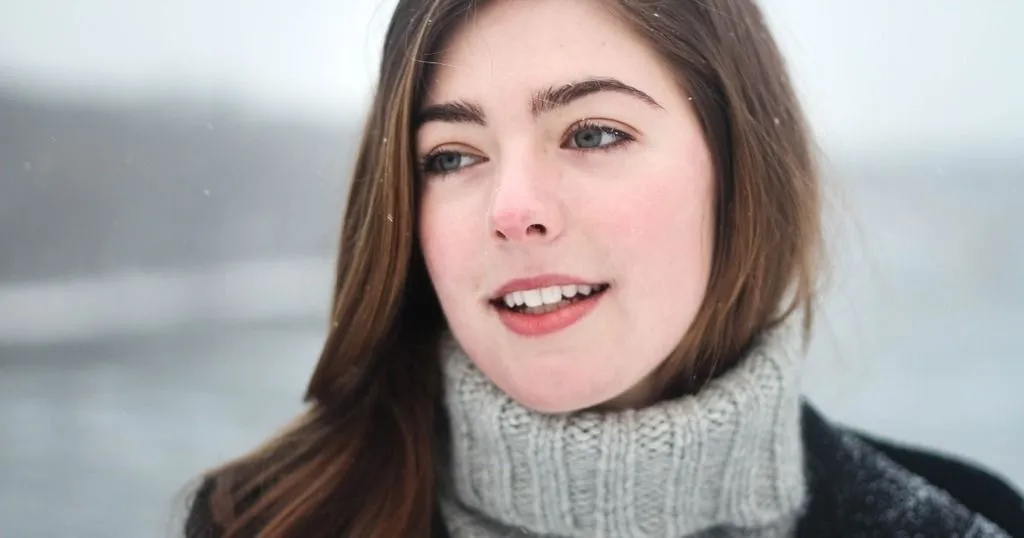
Our emotions come across clear in our facial expressions. Due to this, facial expressions can be used in a wide variety of studies (to identify the reaction of a customer to a product, for instance). It has become a large part of fields such as consumer research and human-computer interaction. FaceReader is an example of an automated tool for facial expression analysis. It provides researchers with an objective assessment of a subject’s emotion based on key points found in the face.
Why this study?
Machine emotion recognition is still fairly new. In this study, Jansen et al. chose to benchmark machine emotion recognition with human performance. They chose to do this based on four reasons.
To start, humans are able to recognize and report the emotions they see in an easy-to-understand way. Also, other uses of artificial intelligence have been benchmarked by human performance. Thirdly, humans are considered superior when it comes to performing mental tasks. Finally, success in artificial intelligence applications is measured by if they are able to beat human performance.
Combining emotion recognition signals
Emotion recognition can be done a variety of different ways, including vision-based, audio-based, and physiology-based. In this experiment, researchers noted that no experiments they found had combined all three of these types to create a multimodal experiment. This could be due to the difficulty of emotion recognition in even unimodal experiments.
Combining modalities was a step forward for the field of emotion studies. In order to create a fundamental benchmark, Janssen et al. collected data that combined the three modalities of signals (speech, vision, and physiological).
Comparing machine emotion recognition with a human benchmark
First part of the experiment: gathering data
There were three parts to this experiment. The first part gathered data through a series of tests involving measuring emotional intensity as the participants recalled certain events in their life. Overall, the neutral condition had a lower emotional intensity than the other conditions.
However, the other conditions did not show a significant difference in emotional intensity between them. The conditions (happy, relaxed, sad, angry, and neutral) did vary some in other areas. Valence was higher in the happy and relaxed conditions and lower in the sad and angry conditions.
The anger condition ended up having the highest arousal levels. Also, the happy and sad conditions were both higher in arousal levels than the neutral and relaxed conditions.
Second part of the experiment: two different languages
The second part of the experiment was actually two separate experiments, one in Dutch and another in American English. In both of these tests, participants watched the recordings made of the first part and described how they imagined the participants felt.
The first experiment used American English speakers to remove the influence of the language structure, as the algorithm in the computers did not use that information. They could rate the emotions of the person speaking merely based on the emotion shown.
The Dutch study was done to see how the emotion recognition task went when the semantic information was also available. With the English speakers, the best emotion recognition performance came when they had the video and audio playing at the same time as opposed to only one of the two.
However, for the Dutch, the context counted and the audio condition resulted in the best emotion recognition.
Third part of the experiment: machine emotion recognition test
Finally, the third part of the experiment was the machine emotion recognition test. Machines were trained based on the data gathered and tested to see how well they would perform. This data came from the video, audio, and physiological modalities measured in the first part.
Researchers were testing to see if the machines could classify the emotions shown into the five classes given. When combining video and audio, the machine did the best, though video alone came close behind. Audio alone didn’t do as well. When adding physiological measures, classification performance was at 76%.
Can machines do a better job than humans?
When looking at a comparison between the results of the humans and the machines, it can be seen that the machines actually performed better. When using video and audio, the machines had a success rate of 65% while humans only had one of 31%. This shows that using machines can be useful for facial expression analysis.
With this information, tools like FaceReader can become valuable for vision-based emotion recognition. It can help automate research on emotion objectively. With non-biased facial expression analysis, expression analysis software can make human-computer interaction research easier and more efficient.
References
- FaceReader, Noldus Information Technology
- Janssen, J.H.; Tacken, P.; Vries, J.J.G. de; Broek, E.L. van den; Westerink, J.H.D.M.; Haselage, P.; IJsselsteijn, W.A. (2013). Machines outperform laypersons in recognizing emotions elicited by autobiographical recollection. Human-Computer Interactions, 28, 479-517.
Related Posts
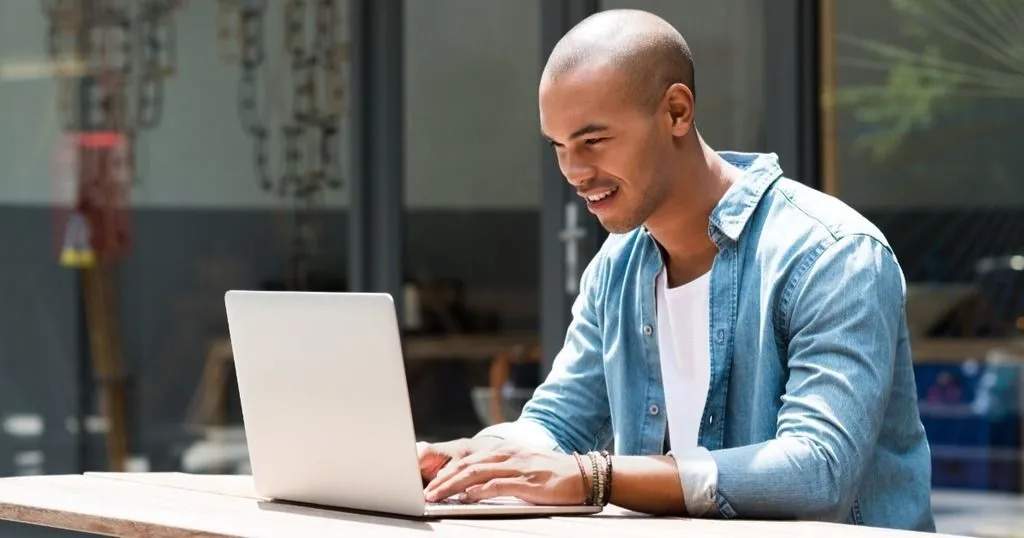
The relevance of emotions on the evaluation of a transactional website
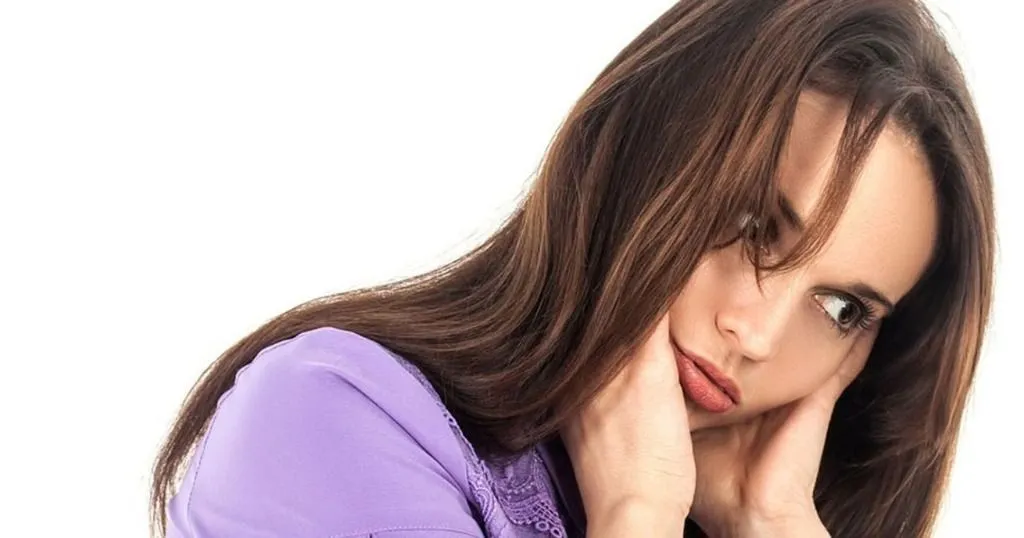
Emotions distract people with eating disorders
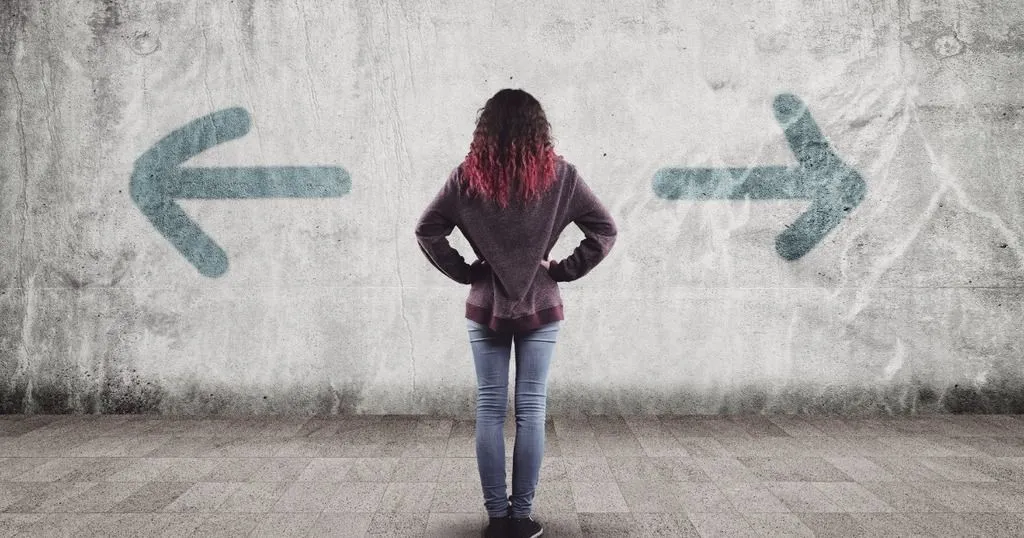