Validating FaceReader's attention detection: a reliable measure using gaze and head pose
Measuring attention is crucial across many fields, from marketing to user experience design and human-computer interaction. Learn more about how we validated custom expressions for attention in FaceReader.
Posted by
Published on
Tue 03 Dec. 2024
Topics
| Attention | Behavioral Psychology | Consumer Behavior | Emotions | FaceReader | Facial Expression Analysis | Measure Emotions | Neuromarketing |
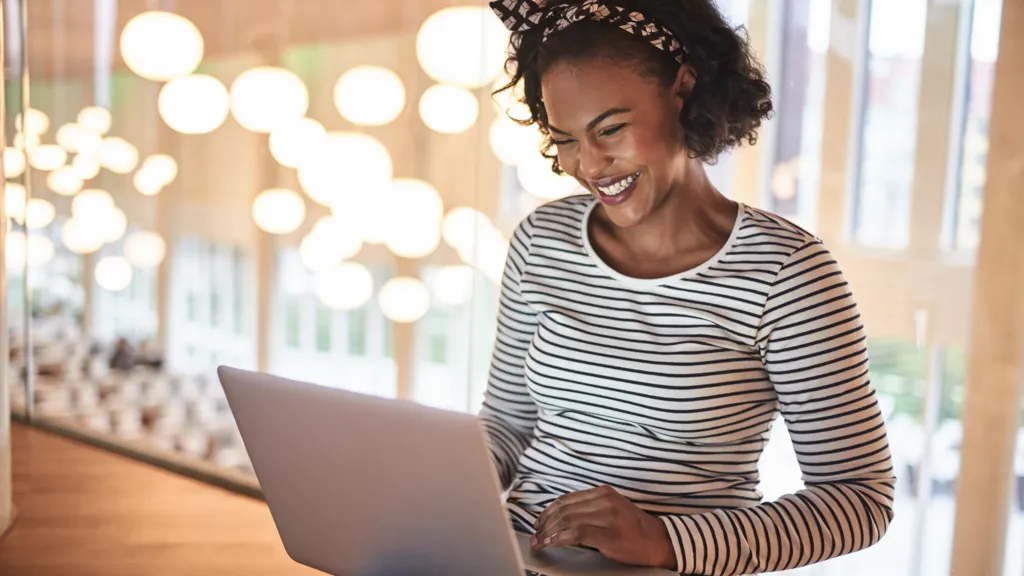
In this blog post, Tess den Uyl, PhD from VicarVision highlights how to use custom expressions to measure attention.
Measuring attention is crucial across many fields, from marketing to user experience design and human-computer interaction.
Learning more about people's attention helps assess the effectiveness of advertisements. For example, people who pay closer attention to ads are more likely to develop positive feelings toward a brand and make repeat purchases.
In user experience research, tracking attention helps to understand what users focus on and how they engage with content. This kind of data helps designers create better interfaces.
Easy gaze tracking with FaceReader
Traditionally, attention is measured using eye trackers. These systems are highly effective. However, they require you to get additional software and also need an extra calibration step. This can be both expensive and time-consuming.
In contrast, facial analysis tools like FaceReader only need a webcam. Easy to access and efficient!
To make sure that FaceReader is effective when measuring attention, we validated several custom expressions we created.
FREE WHITE PAPER: Eye tracking & FaceReader
Download this white paper to learn about:
- Subconscious processes and emotion recognition
- Combining FaceReader™ and eye tracking
- Visualizing and analyzing your data
Studying attention
To create a robust dataset for the validation of attention detection, we used two approaches:
- We selected participants from previous studies
- We recruited additional participants and instructed them to vary their attention, like looking away or checking their phones
This approach gave us a dataset of 101 participants, each providing an average of 1.5 minutes of video footage.
Manual labeling: very time-intensive
We labeled the videos manually, based on a predetermined protocol, resulting in a high inter-rater reliability score of 99%.
Manual labeling involves reviewing videos frame-by-frame to categorize behaviors. This involves a lot of work but is needed to have a robust basis for the comparison of custom expressions later in the experiment.
Different types of attention
Attention is a complex process. It involves both someone's internal cognitive focus and the more visible external signs of attention.
In our study, we focused on external, visible cues like head orientation and gaze direction to assess attention. Results of this type of measurement might differ from a participant's self-reported attention, which often involves internal, subjective elements.
That's why it's so valuable to capture both implicit (visual) and explicit (self-reported) measures of attention. It helps us to discover the full story.
Improving detection of attention
We created several custom expressions to find the most effective combination for attention detection. We mostly focused on gaze and head movements. For each custom expression, we measured:
- Sensitivity: accuracy in detecting attention
- Specificity: accuracy in detecting when attention was absent
Some participants faced challenges, such as poor lighting or partial visibility, which could have influenced results. We used median scores to minimize the effect of these outliers.
Finding the best custom expression
FaceReader's custom expressions use thresholds on different inputs, like facial action units and head orientation. The custom expressions functionality in the Action Unit module allows you to include many simple and complex operations.
Using only head rotation measures—yaw and pitch—resulted in high sensitivity but low specificity. In other words, these measures were accurate in detecting attention, but not in detecting when attention was absent.
When we included gaze direction as well, it significantly improved specificity, making attention classification much more reliable.
In conclusion, this is a well-validated custom expression with a high average accuracy of 0.87, optimizing sensitivity (0.88) and specificity (0.85).
Handling challenging situations
There are challenging scenarios that can complicate attention detection. For example, in cases where participants turned their heads but kept their eyes focused on the screen, a more complex custom expression was needed to maintain accuracy.
Adding additional calculations allowed us to better handle these challenging scenarios. This approach increased complexity but made attention detection more reliable.
Read more blog posts about consumer behavior research.
Applying attention metrics in your own research
Interested in using this attention detection metric for your own research? It’s easy to get started.
If you already use the FaceReader desktop software and have the Action Unit module, you can immediately begin analyzing attention with the included custom expression for attention.
We also offer other pre-made custom expressions—feel free to contact us if you're interested in accessing these!
FaceReader online as a flexible tool
For those who prefer online tools, FaceReader Online is a great option. It’s designed for professionals in fields like advertising, market research, and user experience.
With FaceReader Online, you can evaluate which type of content draws the most attention, making data-driven decisions easier. Want to try for yourself? Request a free trial and get started right away!
Measure attention to improve your research
FaceReader's attention detection offers a reliable way to understand where participants are focusing. Specifically, our study shows that combining gaze and head pose measurements results in the highest accuracy of attention detection.
Whether for marketing, user experience, or psychology research, FaceReader helps you create a more informed view of audience engagement.
Related Posts
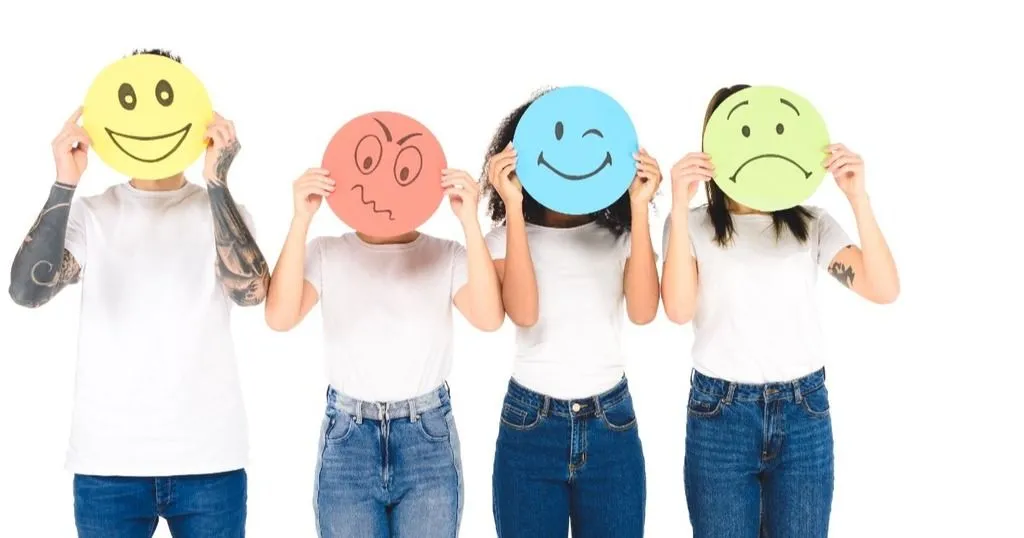
How emotions are made
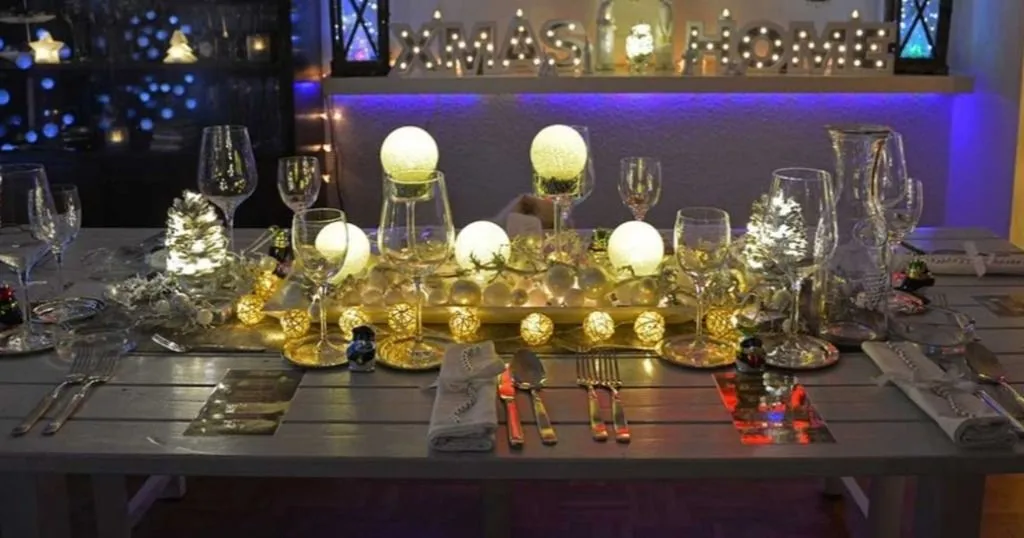
Can facial expressions and emotional reactions predict likeliness to buy?
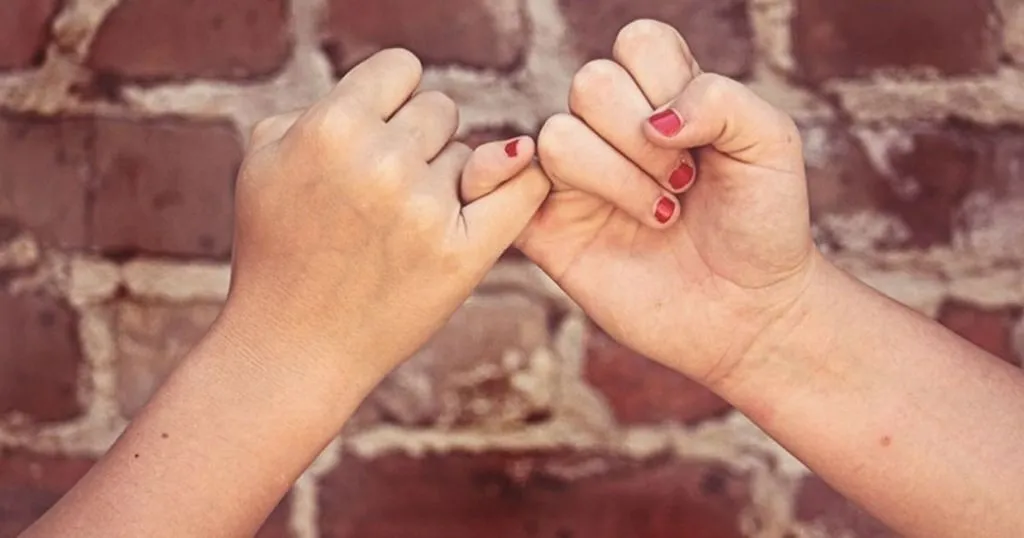